Artificial intelligence (AI) is moving fast, bringing new cybersecurity risks we’re not used to. As AI becomes more common online, it faces many security threats. These include data breaches, attacks, bias, lack of transparency, and more. To keep your data safe from AI threats, we need a complete plan that covers the whole AI process.
AI cyberattacks pose a big risk to our personal data. Machine learning and deep learning make these threats more complex. Hackers might use neural network attacks or automated cybercrimes to get to your private info. It’s important to protect against these risks to keep your data safe and prevent big problems.
The Evolving Landscape of AI Cyber Threats
AI systems are becoming more common in many industries, changing the ai threat landscape. A recent survey found that 74% of companies see ai-powered threats as a big problem. Also, 89% think these threats will keep being a big challenge in the future. Moreover, 56% see ai-powered threats as different from traditional threats.
Understanding the AI Lifecycle and Security Implications
The ai lifecycle has different phases, each with its own ai vulnerabilities. These can be targeted by hackers from design to maintenance. A study by the Department for Science, Innovation and Technology highlighted these risks across the ai lifecycle.
Vulnerabilities Across the AI Lifecycle Phases
In the ai design risks phase, issues can include bad model designs and poor ai training data. During ai development risks, problems can come from using other people’s code. Ai deployment risks bring challenges like integrating systems and setting up security. Lastly, ai maintenance risks include updating software, dealing with model drift, and new threats.
It’s important for companies to tackle these ai lifecycle vulnerabilities. This helps protect their ai systems from cyber threats.
Security Threats: The Risk of AI-Powered Cyberattacks on Personal Data
AI systems are becoming more common, bringing new risks to our personal data. Bad actors can use AI’s weaknesses to launch attacks like data breaches and adversarial attacks. These threats can lead to stolen, changed, or deleted personal info, threatening our privacy and machine learning security risks.
As AI gets better, so do the chances of deep learning cybersecurity vulnerabilities. Criminals can now make complex attacks that are hard to spot and stop. They use AI to make fake emails and malware that change shape, making it tough to keep up with security.
AI models are hard to understand, making security harder. We need a strong plan that uses tech, good data rules, and ongoing checks to protect our data from AI threats.
Data Breaches: The Achilles’ Heel of AI Systems
AI is getting more popular, but it’s facing big security issues. AI uses a lot of data, which can include things like your personal info and company secrets. This data is a big target for hackers who want to break into AI systems.
Securing Training Data: A Crucial Safeguard
Keeping AI training data safe is very important. Hackers are using new tricks to get data from AI models. It’s key to protect this data to keep your personal info safe. Companies need to use strong security steps to protect their AI from these threats.
The ETSI and ENISA are working hard to make AI data safer. They’re creating rules and guidelines to help protect AI data. As AI data privacy risks grow, it’s important for companies and leaders to stay alert and protect your data in AI.
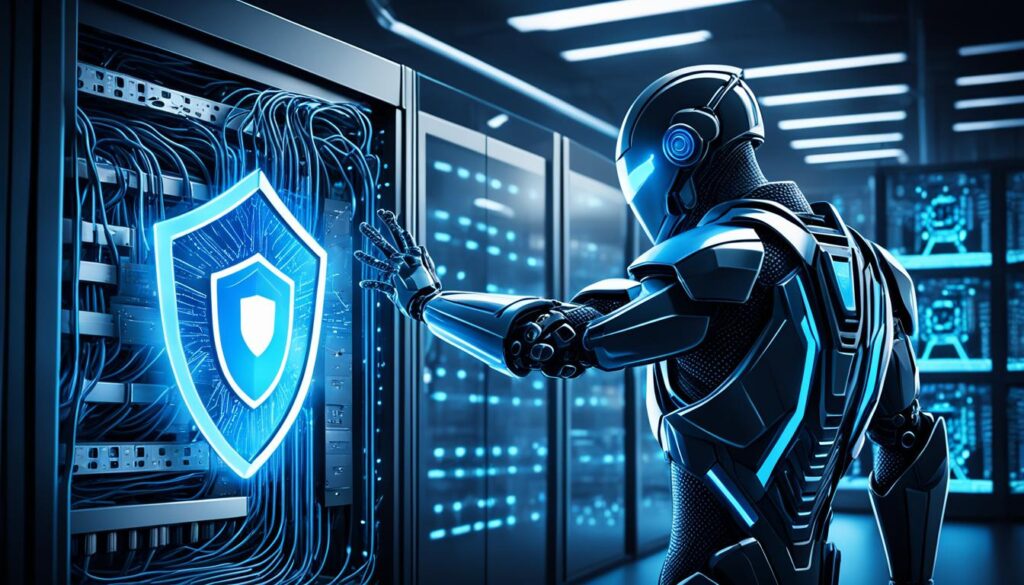
Adversarial Attacks: Exploiting AI Vulnerabilities
Cybercriminals are now using adversarial attacks to mess with AI models and find their weak spots. They train their AI to learn how defensive AI systems work. This lets them spot and use the weaknesses. By adding tiny, hard-to-see changes to data, they can make AI models make wrong choices or miss threats. This makes AI systems less reliable and less secure.
Adversarial attacks are a big threat. They can make AI systems work poorly, leading to wrong data analysis and bad business decisions. This can hurt how well an organization does its job. It’s hard to defend against these attacks because attackers keep changing their ways. This shows we need to keep working on strong security for AI.
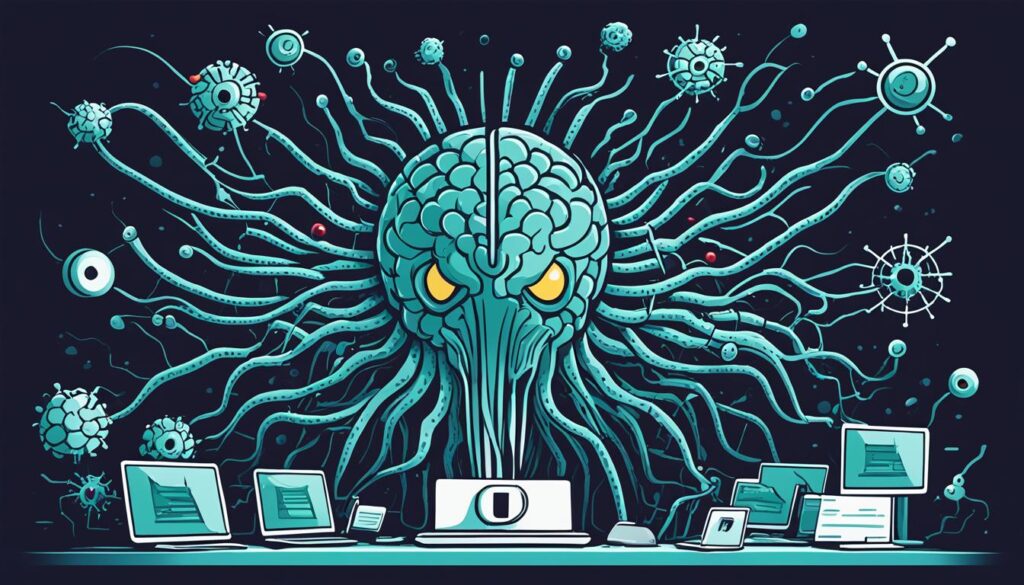
To fight against adversarial attacks, companies need strong security plans for their AI. Using adversarial training, defensive distillation, and feature squeezing can make AI models stronger. These methods help protect AI from being tricked or manipulated. By fixing these weak spots, companies can keep their AI systems safe. This helps protect their data and assets from being fooled by AI attacks.
The Opaque Nature of AI: Lack of Transparency and Bias
AI has changed many industries, but it has also raised a big concern. AI systems are complex, making it hard to know if they have biases or errors. This lack of transparency is a problem because biased training data can make AI models discriminate unfairly.
It’s important to fix these issues of bias and lack of understanding in AI. This ensures AI systems are fair and reliable, especially when they make decisions that affect people’s lives.
Addressing Bias and Discrimination in AI Models
As AI makes more decisions, we must make sure it’s fair and clear. Researchers and developers need to check the training data for biases and fix them. Techniques like model obfuscation, watermarking, and secure enclaves help keep AI models safe from unauthorized access or changes.
There’s a big push for AI to be more transparent. We need AI systems that not only predict well but also explain why they do. By making AI more understandable, we can build trust and ensure it’s fair, reliable, and ethical.
Supply Chain Risks and Third-Party Dependencies
More companies are using third-party AI frameworks and code to build their AI systems. This makes the risk of supply chain problems bigger. AI supply chain risks come from security issues or bad parts in the software. These can be used by attackers to take down the whole AI system.
Managing Security Risks in Open-Source AI Frameworks
Many companies use open-source AI security frameworks like TensorFlow and PyTorch to speed up AI projects. These frameworks have many benefits but also bring third-party AI dependencies that need careful handling. If there are weaknesses in these open-source parts, they can be used by bad actors to attack the AI, risking personal data.
To fight these ai supply chain risks, companies need to have strong security steps throughout the AI’s life. This includes checking third-party parts well, doing regular security checks, and using tools to find and fix AI software weaknesses.
By actively managing third-party AI dependencies and keeping their open-source AI safe, companies can lower the chance of AI-powered cyberattacks. This helps keep their AI systems safe and protects the personal data they handle.
Conclusion
AI’s fast growth brings new cybersecurity risks that need a full approach to tackle. By fixing risks at each AI lifecycle stage, you can make your security stronger. This helps protect your data and keep your AI systems safe from cyber threats.
The AI in cybersecurity market is set to hit about US$102.78 billion by 2032, growing at 19.43% a year. This means we must act fast to tackle these issues. Over 90% of cybersecurity experts worry about AI being used in complex cyberattacks. And 93% think AI threats will hit their companies.
Knowing the risks in the AI lifecycle, from making to using and keeping up, helps you make strong security plans. This way, you can fight against AI cyber threats like data breaches and supply chain attacks. Using the latest research and industry tips, you can keep your data safe and your AI systems strong against new cyber dangers.